AI & Fairness - Case Study on a Grouping Feature
- Sonja Klein
- Mar 4, 2024
- 3 min read
Updated: May 21, 2024
The development of a Grouping Feature aimed to address diverse knowledge levels while ensuring a sense of belonging to a class community. This project was part of a bigger research on "The missing teacher in AI" within the Applied IT Department at the University of Gothenburg.
Background
AI-based personalized learning has been viewed as a disruptive potential in education. The possibility to provide each student with individual learning activities adapted to their own needs and interests, seems to be a solution to the teacher shortage and other educational dilemmas. Nonetheless, schools have not only an educational but also a social responsibility, such as working with others, and discussing ideas while learning social norms and behavior, which can be considered a part of schools as well.
While teachers welcome the chance of individually fitted learning activities for each student, simultaneously they worry about missing opportunities to experience social belonging to a class community. This dilemma becomes especially evident when thinking about group activities within the classroom. Therefore the project aims to develop a function that supports teachers in grouping their students.
Objectives
Develop a feature that is used on a co-creation basis between the teacher & AI.
Give the teacher the last hand, for decisions.
Let AI suggest groups, but allow changes by the teacher.
Ensure fairness in grouping by giving multiple bases to group students.
My Role
I took on the role of the project and product manager of the project team, which consisted of 4 study colleagues of mine and me. This included structuring the overall work processes, documenting, facilitating work processes, and time management.
In my role as user researcher & tester, I was responsible for communicating with other stakeholders, finding and facilitating user research processes, and user tests, as well as evaluating and documenting insights.
Used Methodologies
Human-centered design
We used a human-centered iterative circle within the concept of a double-diamond-shaped design process (as seen in Norman, 2013).
Observations: Personal reflections, interviews, research
To gain a better understanding of the design scenario we discussed our own experiences on social belonging. Since the team was highly international further research and interviews of the Swedish school system were necessary. Therefore, we did a literature review and conducted interviews with experts in the field, as well as teachers from Sweden, India, Lebanon, China & Austria.
Within a later step, I conducted in-person user interviews with three teachers. Based on open design decisions we decided on a semi-structured interview.
Further, we created a questionnaire with the same questions.
Idea Generation: Brainstorming & mind mapping
Together we met for a brainstorming session, where mind maps of ideas were created and afterwards discussed.
Prototyping: Pen & paper sketches, Figma
We started to do pen & paper sketches to find a unified vision of how our product should look like. Doing the sketches this way, not only allowed us to do quick drawings and changes, it also made sure that everyone was able to participate.
We did one round of individual sketching and another one to present and discuss each one. Design decisions were made and documented.
Afterwards, the first clickable Figma sketches were made. The interfaces were distributed and every team member started to transfer the paper sketches into Figma.
User Testing: open discussions & narrative walkthroughs
User Testing was conducted in the form of open discussions with experts & potential users and narrative walkthroughs (see more details here)
Design & Planning
Design Situation
The design situation is set in a Swedish compulsory school classroom where students work individually on tablets, and teachers aim to balance individualized learning with group activities to foster collaboration, motivation, and social belonging. The changing role of teachers from active instructors to learning facilitators poses a challenge in understanding students' progress and grouping them effectively.
Challenges & Solutions
All challenges were based on the research & specifics of the design situation.
Aspect of Grouping
Teachers' Need for Versatility
Teachers want an adjustable grouping tool for various activities, including strengthening knowledge on specific topics or fostering social skills.
Functions: Choose Group Size, Add New Group, Pick Whom to Group
Grouping Options
Equal Distribution of Knowledge and Skill:
Teachers aim to group students based on knowledge levels to avoid disparities.
Functions: grouping by the same level, mixed level, random, and interest-based
Learning from Each Other
Varied groups are vital for learning from peers. Teachers may intentionally group students of mixed levels, especially for activities beyond core content.
Consideration of Emotional and Social Aspects
Personal conflicts and relationships influence group dynamics.
Functions: grouping based on emotional, social status and interests
Transparency & Avoiding
Sharing Workload
Teachers want to share workload responsibilities, and in classrooms with multiple teachers, workload distribution is essential
Function: assign a specific teacher to the group
Avoiding Repetition in Groups
Teachers worry about having the same groups repeatedly.
Function: provide options to continue with old groups or build new ones
Decision-making & Flexibility
Understanding the Basis of Grouping
Teachers express concerns about the transparency of AI-based grouping decisions.
Function: show clear information on what the AI is doing
Teacher's Final Call
Teachers insist on having the final say in grouping decisions, emphasizing their responsibility to students.
Function: allow teachers to check and approve groups
Last-Minute Changes
Teachers want the flexibility to make last-minute changes. They appreciate AI suggestions but need the ability to modify groups based on their experience.
Function: making changes available in all stages, confirming AI work by teachers
Prototypes
These mockups were built on the assumption of a functioning personalized learning system, that features each student with individual learning paths, small quizzes as well as questions on how hard the exercises were for them.
The feature is not specified to a subject, because this tool is a stand-alone feature. Using it in different situations would make it even better because the groups might look different then.
The teacher can pick a topic, the kind of exercise, and a specific task that has been suggested by AI. The workflow of picking the topic first and the groups afterward was chosen, because teachers said that this would reflect their procedure when they want students to learn something specific. However, if the product would be developed further it would make sense to add a reversed workflow that would start with grouping students, before picking a specific topic.
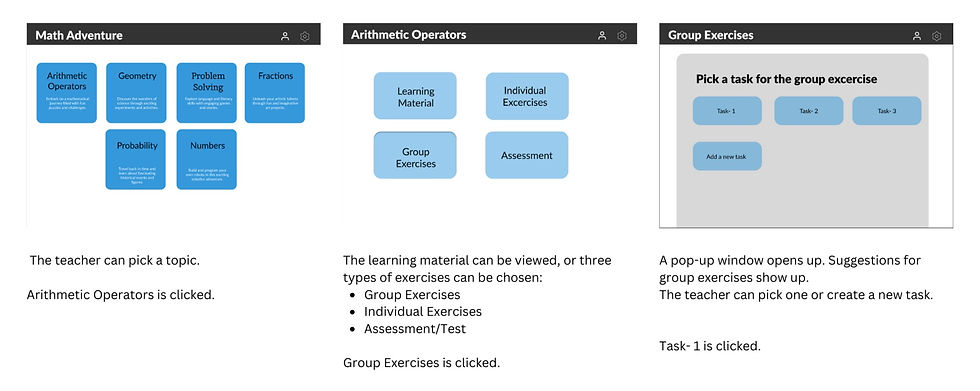
Grouping features
There are three possibilities for grouping students:

Knowledge-based
Grouping based on the same level or mixed level of knowledge
Addressing teachers' concerns about learning from each other
Interest-based
Grouping based on students shared interests
Motivating them with topics they find intrinsically interesting
Random
Random grouping, favored by teachers for equality and speed
Teachers can adapt and make changes to the randomly generated groups
Adaptions by the Teacher
Three possible adaptions by teachers were defined and prototyped in detail:
Picking students
Teachers can manually select students for group activities, syncing with the attendance record to streamline the process.
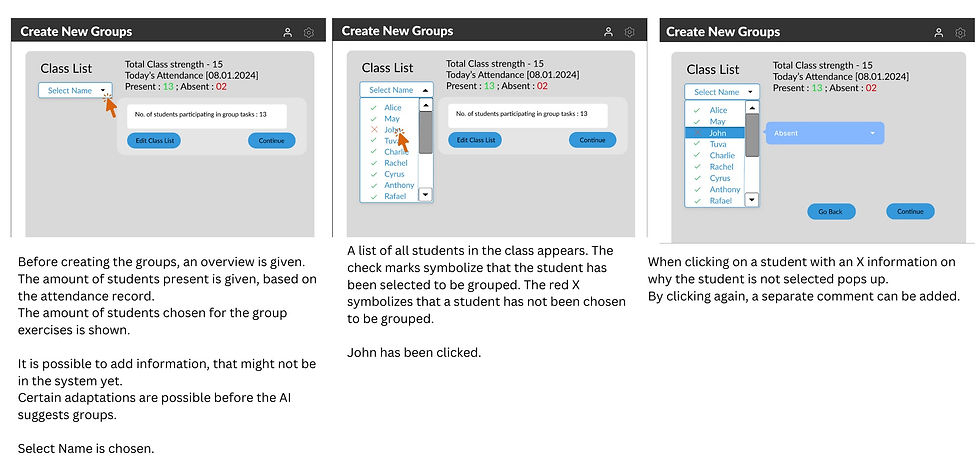
Adding no-gos
Teachers can specify dependencies to avoid pairing certain students, reflecting real-world considerations
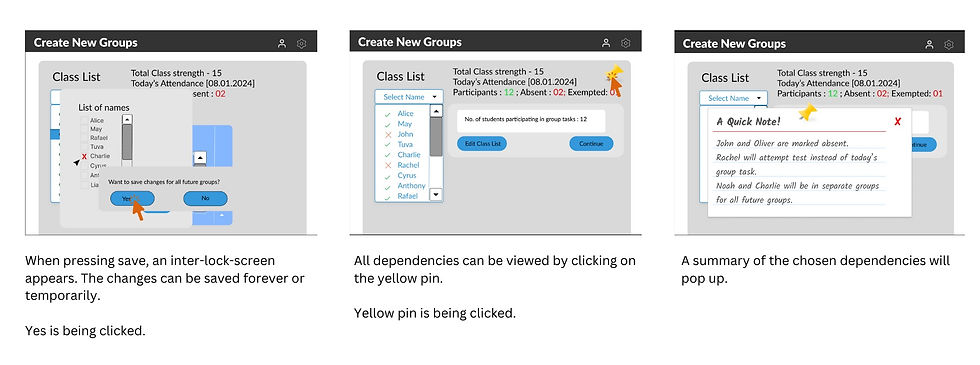
Editing AI-based group suggestions
AI suggests groups based on entered and retrieved data, allowing manual changes
Teachers can save adaptations temporarily or long-term to train the AI
User - Testing
The user testing showed valuable insights for further refinement (see more here).
Interface Improvements
Suggestions for clearer wording
A home button
Drag-and-drop options for quick changes
Enhanced Functionality
Considerations for additional features like social groups, empowerment buttons, and a mix of random and knowledge-based grouping
Tool Efficiency
Emphasis on the tool being faster than manual grouping
Facilitating last-minute changes.
Conclusion
The project successfully navigated through iterative design cycles, addressing the complex challenges of grouping students in a personalized learning environment. The prototype presented features that catered to teachers' needs, fostering a sense of transparency, flexibility, and control. While recognizing open questions for future research, the design solution aimed to contribute to ongoing efforts in enhancing classroom dynamics through AI-powered tools.
Main Learnings & Competences
Competences developed within this project:
Creating mock-ups in Figma
Facilitating User interviews & tests
Developing an innovative product from scratch
Working with an intercultural team from China, Lebanon, Sweden & India
Managing a team in person & online
Presenting prototypes to experts and possible users
Comments